AbstractConsidering the fact that points of interest on 3D shapes can be discriminated from a geometric perspective, it is reasonable to map the geometric signature of a point p to a probability value encoding to what degree p is a point of interest, especially for a specific class of 3D shapes. Based on the observation, we propose a three-phase algorithm for learning and predicting points of interest on 3D shapes by using multiple feature descriptors. Our algorithm requires two separate deep neural networks (stacked auto-encoders) to accomplish the task. During the first phase, we predict the membership of the given 3D shape according to a set of geometric descriptors using a deep neural network. After that, we train the other deep neural network to predict a probability distribution defined on the surface representing the possibility of a point being a point of interest. Finally, we use a manifold clustering technique to extract a set of points of interest as the output. Experimental results show superior detection performance of the proposed method over the previous state-of-the-art approaches.
PublicationZhenyu Shu, Shiqing Xin, Xin Xu, Ligang Liu, Ladislav Kavan. Detecting 3D Points of Interest Using Multiple Features and Stacked Auto-encoder. IEEE Transactions on Visualization and Computer Graphics, 2018. 
Links and DownloadsPaper
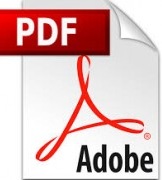 | | BibTeX
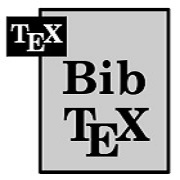 |
AcknowledgementsThe authors would like to thank the anonymous reviewers
for their valuable comments and suggestions. This work is
supported by National Natural Science Foundation of China
(11226328, 61672482, 11626253, 61772016, 61572022), National
Science Foundation (IIS-1617172, IIS-1622360), Natural
Science Foundation of Zhejiang Province (LY17F020018),
Ningbo Leader and Top-notch Talent Training Project,
Ningbo Innovative Team: The intelligent big data engineering
application for life and health (2016C11024), the
Fundamental Research Funds of Shandong University, and
the Open Project Program of the State Key Lab of CAD and
CG (Grant No. A1702), Zhejiang University.  |